Open Access
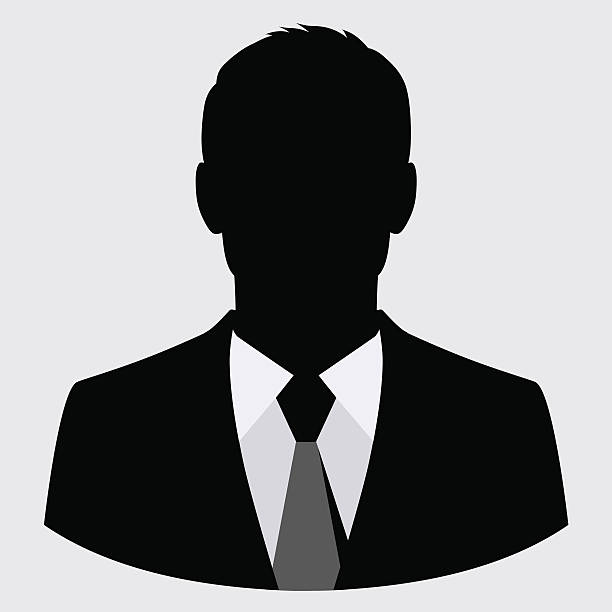
Amrutha L.,
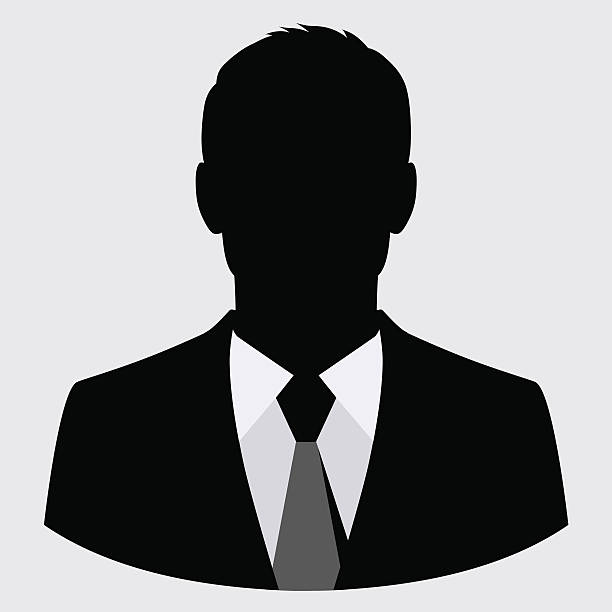
Rachita R,
- Student Department of Computer Sciences, Global Academy of Technology Bangalore India
- Student Department of Computer Sciences, Global Academy of Technology Bangalore India
Abstract
Heart disease which can also be referred to as the cardiovascular disease is one of the raising concerns in today’s world. It is one of the major health problems causing death among humans irrespective of the age group and therefore has made it necessary to look into different medical factors that are required to predict the same in advance using the collected historical datasets of various patients. Thus we have used various machine learning algorithms to predict the potential of person, to suffer from a heart disease with high precision and reliability so that we can admonish the patient in advance and take the required precautionary measures. In this paper an existing heart disease dataset accessible from the UCI Machine Learning Repository is used as the primary dataset. The experimental analysis and comparative study between different algorithms, helps in deciding the best suitable algorithm for the given problem statement by using results obtained which are very competitive and can be used for identification and treatment. The proposed work predicts the probability of heart condition and ranks the patient’s risk level based on different supervised learning algorithms such as K-Neighbors, AdaBoost, Gradient Boosting. The test results portrays that K- neighbors has the highest accuracy score of 91% when compared with other algorithms.
Keywords: Heart disease prediction, algorithms, machine learning, CNN, KNN
References
1. Apurb Rajdhan, Milan Sai, Avi Agarwal, Dundigalla Ravi. Heart Disease Prediction using Machine Learning. International Journal of Engineering Research & Technology (IJERT).2020;9 (4)
2. Rindhe BU, Ahire N, Patil R, Gagare S, Darade M. Heart Disease Prediction Using Machine Learning. Heart Disease. 2021 May;5(1).
3. Jindal H, Agrawal S, Khera R, Jain R, Nagrath P. Heart disease prediction using machine learning algorithms. InIOP conference series: materials science and engineering 2021 (Vol. 1022, No. 1, p. 012072). IOP Publishing.
4. Shah D, Patel S, Bharti SK. Heart disease prediction using machine learning techniques. SN Computer Science. 2020 Nov;1(6):1-6.
5. Bharti R, Khamparia A, Shabaz M, Dhiman G, Pande S, Singh P. Prediction of heart disease using a combination of machine learning and deep learning. Computational intelligence and neuroscience. 2021 Jul 1;2021.
6. Golande A, Pavan Kumar T. Heart disease prediction using effective machine learning techniques. International Journal of Recent Technology and Engineering. 2019 Jun;8(1):944-50.
7. Beyene C, Kamat P. Survey on prediction and analysis the occurrence of heart disease using data mining techniques. International Journal of Pure and Applied Mathematics. 2018 Jan;118(8):165- 74.
8. Soni J, Ansari U, Sharma D, Soni S. Predictive data mining for medical diagnosis: An overview of heart disease prediction. International Journal of Computer Applications. 2011 Mar 8;17(8):43-8.
9. Seckeler MD, Hoke TR. The worldwide epidemiology of acute rheumatic fever and rheumatic heart disease. Clinical epidemiology. 2011;3:67.
10. Mohan, Senthilkumar, Chandrasegar Thirumalai, and Gautam Srivastava, “Effective heart disease prediction using hybrid machine learning techniques” IEEE Access 7 (2019): 81542-81554.
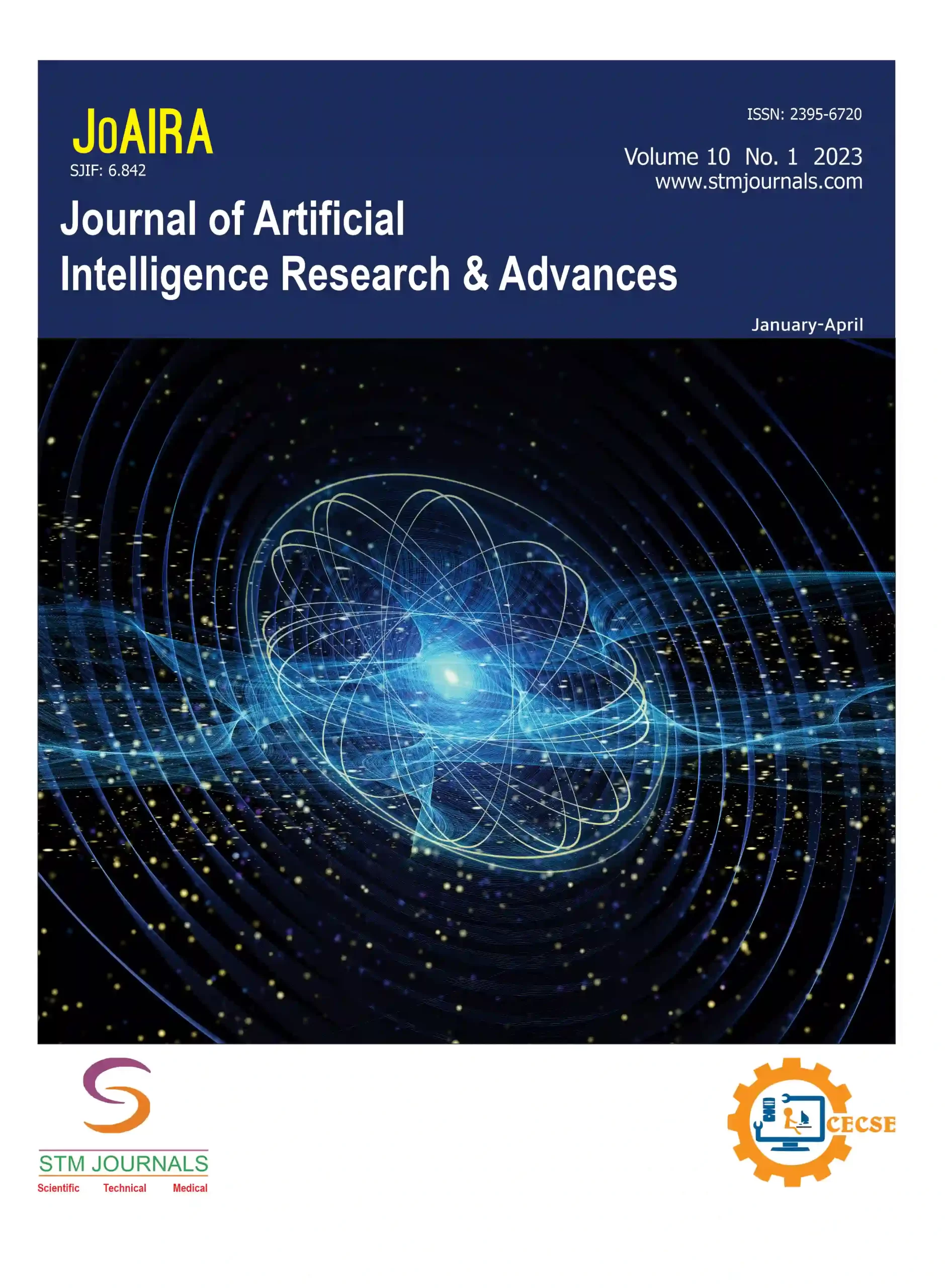
Journal of Artificial Intelligence Research & Advances
Volume | |
Received | August 10, 2022 |
Accepted | August 18, 2022 |
Published | January 30, 2023 |