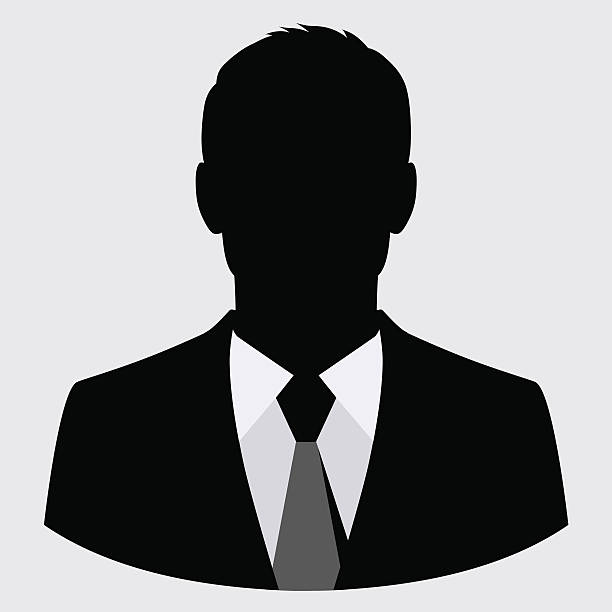
Syed Matheen Pasha
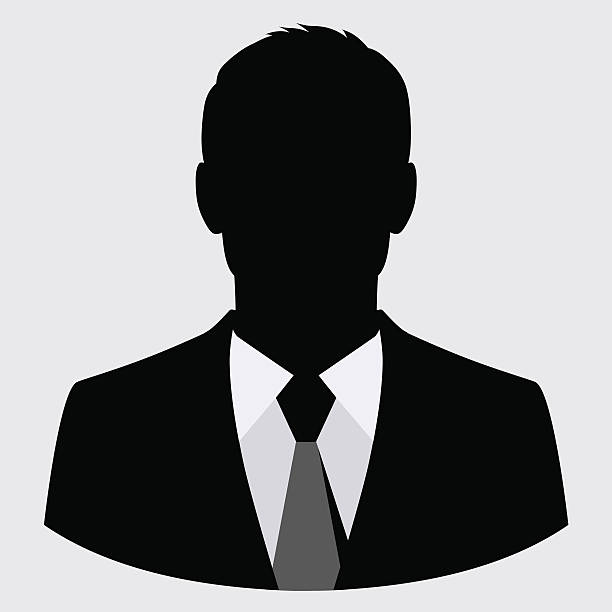
Shanta Kumar Patil
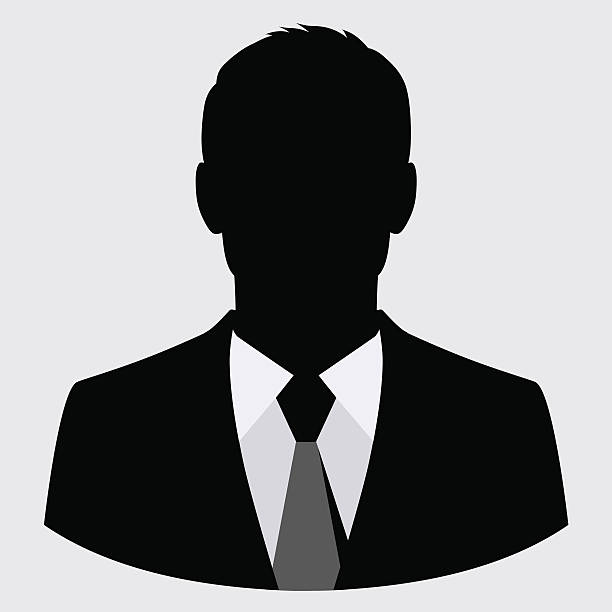
N. Ashok Kumar
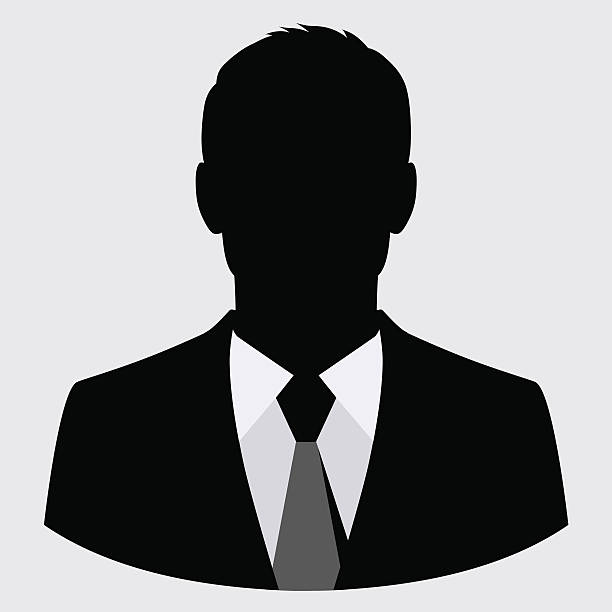
G. Likeeth
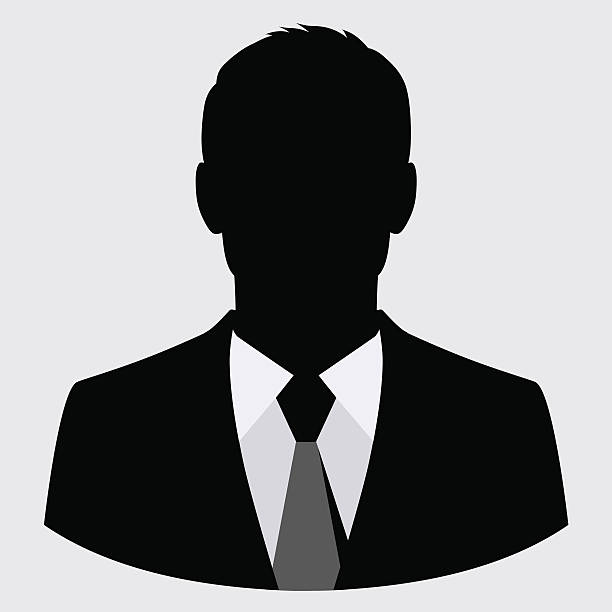
Hemanth Kumar S
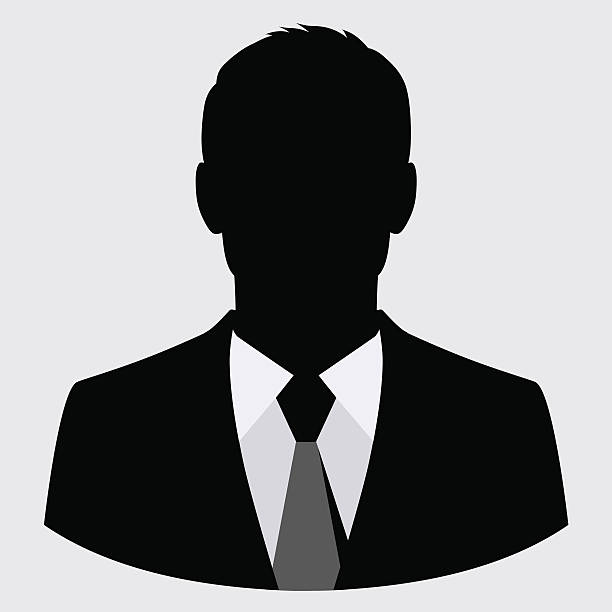
Karthik Reddy
- Professor Department of Computer Science and Engineering, Sai Vidya Institute of Technology, Bangalore Karnataka India
- Professor Department of Computer Science and Engineering, Sai Vidya Institute of Technology, Bangalore Karnataka India
- Student Department of Computer Science and Engineering, Sai Vidya Institute of Technology, Bangalore Karnataka India
- Student Department of Computer Science and Engineering, Sai Vidya Institute of Technology, Bangalore Karnataka India
- Student Department of Computer Science and Engineering, Sai Vidya Institute of Technology, Bangalore Karnataka India
- Student Department of Computer Science and Engineering, Sai Vidya Institute of Technology, Bangalore Karnataka India
Abstract
Any physical object that can be identified by an IP address to permit data transmission over a network can become a part of the Internet of Things by installing electronic gear like sensors, networking hardware, and software. Improved connectivity for a variety of devices, services, protocols, and applications is provided via the Internet of Things (IoT). Its heterogeneous character further defines it. IoT has proven effective not just in homes and smart cities. In Urban regions produce a lot of solid rubbish, which is composed of many materials like paper, plastic, metal, glass, and organic waste. For waste management to be effective, these products must be treated separately. To solve this problem, governments have passed laws mandating the classification of waste into dry and wet categories. Following these recommendations not only helps with efficient waste management but also drastically lowers the cost of trash segregation, freeing up money for further waste treatment expenditures. This study suggests a way to classify waste as dry or moist only by looking at pictures of it. The proposed application allows local government bodies to upload images of trash cans for inspection, which expedites the process. Machine learning techniques are employed to detect waste, potentially facilitating future examination of waste disposal methods in different locations. This study can help guide targeted awareness initiatives to improve waste disposal methods.
Keywords: Waste analysis, machine learning, artificial intelligence, internet of things (IoT), IP addresses.
[This article belongs to Journal of Alternate Energy Sources & Technologies(joaest)]
Browse Figures
References
- Shaikh F, Kazi N, Khan F, Thakur Z. Waste profiling and analysis using machine learning. In2020 second international conference on inventive research in computing applications (ICIRCA) 2020 Jul 15 (pp. 488-492). IEEE.
- Waste Management Initiative In India For Human Well Being “ European scientific journals june 2015”
- G, and Y. Tagawa, “ Electron spectroscopy studies on magneto-optical media and plastic substrate interface,” IEEE Transl. J. Magn. Japan, vol. 2, pp. 740–741, August 1987 [Digests 9th Annual Conf. Magnetics Japan, p. 301, 1982].
- Young, The Technical Writer’s Handbook. Mill Valley, CA: University Science, 1989.
- Anh Khoa T, Phuc CH, Lam PD, Nhu LM, Trong NM, Phuong NT, Dung NV, Tan-Y N, Nguyen HN, Duc DN. Waste management system using IoT-based machine learning in university. Wireless Communications and Mobile Computing. 2020 Feb 27;2020:1-3.
- Wulansari A, Setyanto A, Luthfi ET. Systematic literature review of waste classification using machine learning. JOURNAL OF INFORMATICS AND TELECOMMUNICATION ENGINEERING. 2022 Jan 26;5(2):405-13.
- Sami KN, Amin ZM, Hassan R. Waste management using machine learning and deep learning algorithms. International Journal on Perceptive and Cognitive Computing. 2020 Dec 14;6(2):97-106.
- Uganya G, Rajalakshmi D, Teekaraman Y, Kuppusamy R, Radhakrishnan A. A novel strategy for waste prediction using machine learning algorithm with IoT based intelligent waste management system. Wireless Communications and Mobile Computing. 2022 Oct;2022.
- Gary Thung and Mindy Yang, “ Classification of Trash for Recyclability Status,” CS 229, Stanford University, 2016.Available: https://github.com/garythung/trashnet
- Dubey S, Singh P, Yadav P, Singh KK. Household waste management system using IoT and machine learning. Procedia Computer Science. 2020 Jan 1;167:1950-9.
- Xia W, Jiang Y, Chen X, Zhao R. Application of machine learning algorithms in municipal solid waste management: A mini review. Waste Management & Research. 2022 Jun;40(6):609-24.
- Meza JK, Yepes DO, Rodrigo-Ilarri J, Cassiraga E. Predictive analysis of urban waste generation for the city of Bogotá, Colombia, through the implementation of decision trees-based machine learning, support vector machines and artificial neural networks. Heliyon. 2019 Nov 1;5(11).
- Flores MG, Tan J. Literature review of automated waste segregation system using machine learning: A comprehensive analysis. International journal of simulation: systems, science and technology. 2019 Jul 2;11.
- Rutqvist D, Kleyko D, Blomstedt F. An automated machine learning approach for smart waste management systems. IEEE transactions on industrial informatics. 2019 May 8;16(1):384-92.
- Kannangara M, Dua R, Ahmadi L, Bensebaa F. Modeling and prediction of regional municipal solid waste generation and diversion in Canada using machine learning approaches. Waste management. 2018 Apr 1; 74:3-15.
- [Kontokosta CE, Hong B, Johnson NE, Starobin D. Using machine learning and small area estimation to predict building-level municipal solid waste generation in cities. Computers, Environment and Urban Systems. 2018 Jul 1; 70:151-62.
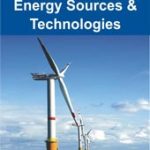
Journal of Alternate Energy Sources & Technologies
Volume | 15 |
Issue | 01 |
Received | May 9, 2024 |
Accepted | May 18, 2024 |
Published | May 28, 2024 |