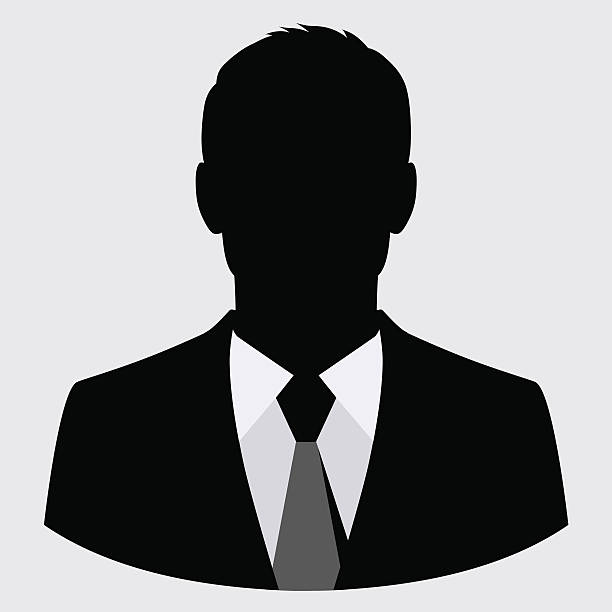
Manas Kumar Yogi,
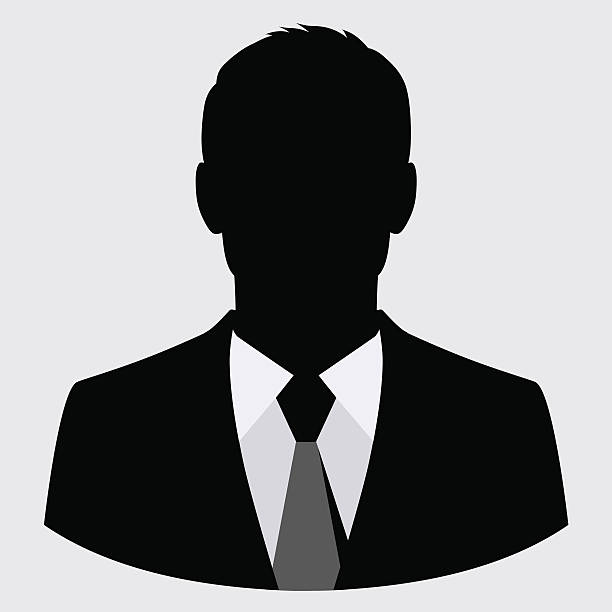
Yamuna Mundru,
- Assistant Professor Department of computer science and engineering, Pragati Engineering College (Autonomous) Andhra Pradesh India
- Assistant Professor CSE-AI& ML Department, Pragati Engineering College (Autonomous) Andhra Pradesh India
Abstract
The ever-evolving landscape of cybersecurity necessitates continuous advancements in malware analysis techniques. This study explores the deployment of the Grey Wolf Optimizer (GWO) algorithm as a novel bio-inspired optimization mechanism to address the challenges posed by modern malware threats. The primary objective is to enhance various facets of malware analysis, including feature selection, parameter optimization, and the overall efficacy of malware detection models. The study begins by introducing the GWO algorithm, elucidating its fundamental principles and mechanisms. It subsequently details how GWO can be effectively adapted to the realm of malware analysis, emphasizing its role in improving the selection of discriminative features and optimizing the parameters of machine learning models. In pursuit of empirical validation, a comprehensive experimental setup is presented, featuring diverse malware datasets, well-defined evaluation metrics, and baseline models for performance comparison. The experimental results unveil compelling findings: the deployment of GWO consistently yields substantial enhancements in the accuracy and resilience of malware detection systems. Notably, GWO exhibits remarkable effectiveness in addressing the dynamic and polymorphic nature of contemporary malware, making it a valuable asset for real-time threat identification. The significance of these discoveries has broad implications for both research and practical use in the realm of cybersecurity. The deployment of GWO emerges as a potent strategy for fortifying the capabilities of malware detection systems, rendering them more adaptive and proficient in discerning emerging threats. Furthermore, this study underscores the importance of exploring innovative, nature-inspired approaches, such as GWO, to keep pace with the ever-shifting landscape of cyber threats. In conclusion, this study illuminates the promising potential of the Grey Wolf Optimizer (GWO) mechanism as a transformative tool for malware analysis, ushering in a new era of precision and efficiency in the ongoing battle against malware-induced vulnerabilities.
Keywords: Grey Wolf Optimizer, Malware, Virus, Trojan, Threat, Security
[This article belongs to International Journal of Wireless Security and Networks(ijwsn)]
References
1. Or-Meir Ori, et al. Dynamic malware analysis in the modern era—A state of the art survey. ACM Comput Surv (CSUR). 2019; 52(5): 1–48.
2. Egele Manuel, et al. A survey on automated dynamic malware-analysis techniques and tools. ACM Comput Surv (CSUR). 2008; 44(2): 1–42.
3. Gandotra Ekta, Divya Bansal, Sanjeev Sofat. Tools & Techniques for Malware Analysis and Classification. International Journal of Next-Generation Computing (IJNGC). 2016; 7(3): 176–197.
4. Mohanta Abhijit, Anoop Saldanha. Malware Analysis and Detection Engineering: A Comprehensive Approach to Detect and Analyze Modern Malware. New York, NY, USA: Apress; 2020.
5. Wagner Markus, et al. A survey of visualization systems for malware analysis. Eurographics Conference on Visualization (EuroVis). 2015; 105–125.
6. Mirjalili Seyedali, Seyed Mohammad Mirjalili, Andrew Lewis. Grey wolf optimizer. Adv Eng Softw. 2014; 69: 46–61.
7. Faris Hossam, et al. Grey wolf optimizer: a review of recent variants and applications. Neural Comput Appl. 2018; 30(2): 413–435.
8. Al-Tashi Qasem, et al. A review of grey wolf optimizer-based feature selection methods for classification. In Book: Evolutionary Machine Learning Techniques: Algorithms and Applications. Singapore: Springer; 2020; 273–286.
9. Gupta Shubham, Kusum Deep. A novel random walk grey wolf optimizer. Swarm Evol Comput. 2019; 44: 101–112.
10. Güllü Merve, Necattin Barişçi. Android Malware Classification with Gray Wolf Optimization Algorithm and Deep Neural Network Hybrid Approach. 2022 IEEE 30th Signal Processing and Communications Applications Conference (SIU). 2022.
11. Qaddoura Raneem, et al. A classification approach based on evolutionary clustering and its application for ransomware detection. Evolutionary Data Clustering: Algorithms and Applications. Singapore: Springer; 2021; 237–248.
12. Alzaqebah Abdullah, et al. A modified grey wolf optimization algorithm for an intrusion detection system. Mathematics. 2022; 10(6): 999.
13. Jaber Aws Naser, Lothar Fritsch, Hårek Haugerud. Improving phishing detection with the grey wolf optimizer. 2022 IEEE International Conference on Electronics, Information, and Communication (ICEIC). 2022; 1–6.
14. Almazini Hussein, Ku Ruhana Ku-Mahamud. Grey Wolf Optimization Parameter Control for Feature Selection in Anomaly Detection. Int J Intell Eng Syst. 2021; 14(2): 474–482.
Volume | 01 |
Issue | 02 |
Received | September 11, 2023 |
Accepted | September 22, 2023 |
Published | September 25, 2023 |